Synthetic Data Is a Dangerous Teacher
Synthetic Data Is a Dangerous Teacher
With the rise of artificial intelligence…
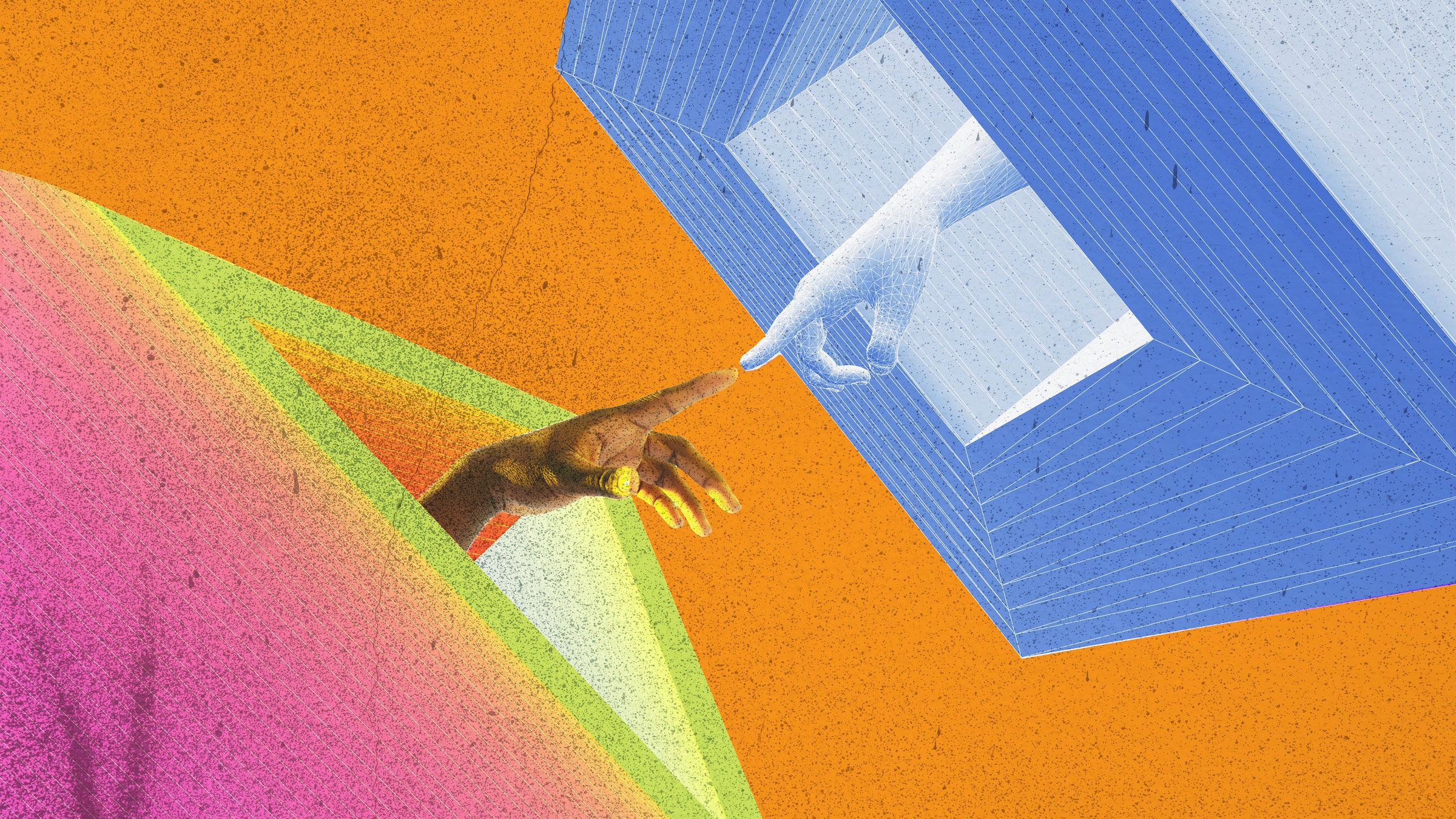
Synthetic Data Is a Dangerous Teacher
With the rise of artificial intelligence and machine learning, the use of synthetic data has become more prevalent in training models. While synthetic data can be useful in certain scenarios, it is important to recognize its limitations and potential dangers.
One major issue with synthetic data is that it may not accurately reflect real-world scenarios. This can lead to models that are biased or perform poorly when faced with new, unseen data.
Additionally, synthetic data can inadvertently reinforce existing biases in a dataset. If the synthetic data is generated based on a biased dataset, it can perpetuate those biases in the model.
Another concern is the potential for synthetic data to be used maliciously. By creating synthetic data that closely mimics real data, attackers could potentially manipulate models and cause serious harm.
Furthermore, relying too heavily on synthetic data can lead to a false sense of security. Models trained on synthetic data may perform well in a controlled environment, but struggle when faced with the complexities of the real world.
It is important for researchers and practitioners to approach the use of synthetic data with caution and ensure that it is being used thoughtfully and responsibly.
In conclusion, while synthetic data can be a valuable tool in training machine learning models, it is crucial to understand its limitations and potential risks. Synthetic data is a dangerous teacher that must be used wisely to avoid unintended consequences.